AIOps / NoOps
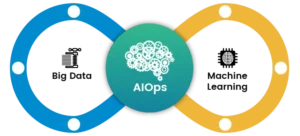
AIOps, or Artificial Intelligence for IT Operations, is an emerging field that leverages machine learning and artificial intelligence to automate and streamline IT operations. In today’s fast-paced and complex IT landscape, AIOps is becoming increasingly popular as organizations seek to improve their operational efficiency, reduce downtime, and enhance the overall user experience.
What is AIOps?
AIOps is an approach that uses artificial intelligence and machine learning to analyze and automate IT operations tasks. This includes tasks such as monitoring, incident management, root cause analysis, and predictive analytics. AIOps enables organizations to detect, diagnose, and resolve issues faster and more accurately, and also provides insights into patterns and trends that can help prevent issues from occurring in the first place.
How does AIOps work?
AIOps uses various data sources, such as logs, metrics, and events, to train machine learning algorithms that can detect patterns and anomalies. These algorithms can then be used to predict potential issues and automate the resolution process. For example, an AIOps system can use machine learning algorithms to analyze log files and identify patterns that indicate a potential issue. It can then automatically initiate a remediation process to resolve the issue before it impacts the end-user.
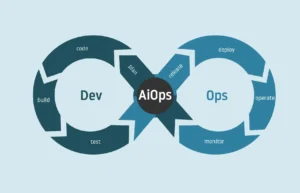
Benefits of AIOps
- Faster issue resolution: AIOps can detect and diagnose issues faster than traditional manual methods, reducing downtime and minimizing the impact on end-users.
- Improved efficiency: AIOps can automate routine tasks, freeing up IT staff to focus on more strategic initiatives and improving overall operational efficiency.
- Enhanced user experience: AIOps can help to prevent issues before they occur, improving the overall user experience and reducing the risk of downtime or service disruption.
- Predictive analytics: AIOps can use predictive analytics to identify potential issues before they occur, enabling IT teams to take proactive measures to prevent them.
- Scalability: AIOps can scale to accommodate large amounts of data, enabling organizations to monitor and manage their IT infrastructure more effectively.
Challenges of AIOps
Implementing an AIOps system can be a complex and challenging process, requiring significant investment in technology, data integration, and expertise. Some of the key challenges associated with AIOps include:
- Data integration: AIOps requires the integration of data from various sources, which can be a complex and time-consuming process.
- Data quality: AIOps systems rely on high-quality data to provide accurate insights and predictions. Ensuring data quality can be a challenge, as data sources may be inconsistent or incomplete.
- Expertise: AIOps requires expertise in data science and machine learning, which can be difficult to find and expensive to hire.
- Change management: Implementing an AIOps system requires significant changes to existing IT processes and workflows, which can be challenging to manage and may require significant cultural change.
AIOps represents a significant opportunity for organizations to improve their operational efficiency and reduce downtime. By leveraging machine learning and artificial intelligence to automate IT operations tasks, AIOps can help organizations to detect and resolve issues faster, improve the overall user experience, and reduce the risk of downtime and service disruption. However, implementing an AIOps system can be a complex and challenging process, requiring significant investment in technology, data integration, and expertise. To be successful with AIOps, organizations must carefully consider their objectives, strategy, and implementation approach, and work closely with experts in data science and machine learning to achieve their goals.